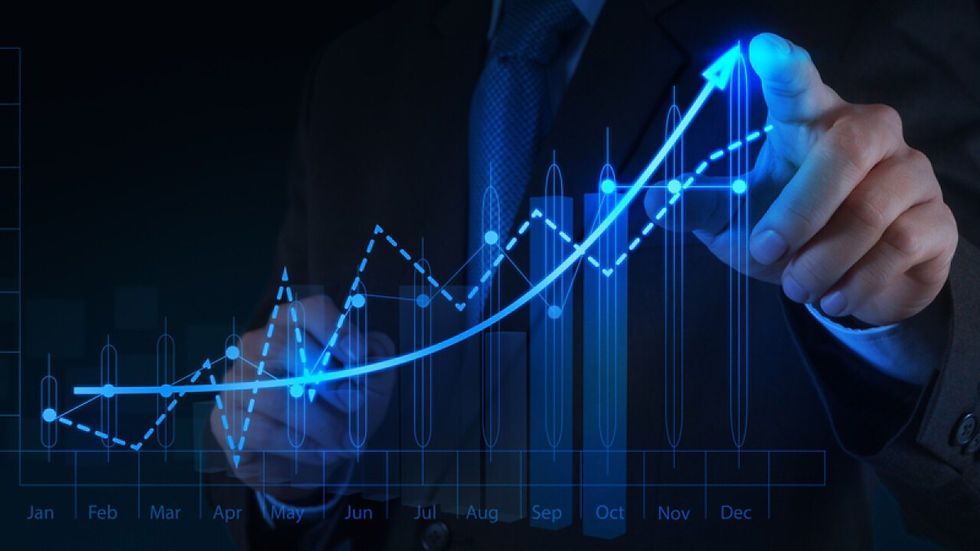
Trend analysis plays a crucial role in various fields, transforming raw data into meaningful insights. Whether in finance, healthcare, or environmental studies, regression analysis remains a key tool for deciphering trends and making informed decisions. This post highlights the importance of regression, its methods, applications, and the implications in multiple industries.
Understanding Regression in Trend Analysis
At its essence, regression analysis is a statistical method that investigates the relationship between a dependent variable and one or more independent variables. It allows analysts to understand how changes in independent variables can influence the dependent variable.
In trend analysis, regression is invaluable for identifying patterns over time and predicting future values based on historical data. For instance, a company can analyze how advertising spend impacts product sales over several months, using regression to forecast future sales based on advertising trends.
Types of Regression Techniques
There are different regression techniques to suit various scenarios. Knowing these methods is vital for selecting the proper approach for specific data.
1. Linear Regression
Linear regression models relationships as a straight line, making it useful when a linear relationship exists between the variables.
Example
For example, a study examining the connection between daily temperatures and ice cream sales can use linear regression to show that as temperatures rise by 10 degrees, ice cream sales might increase by 20%.
2. Multiple Regression
Involving multiple independent variables, multiple regression acknowledges that several factors can impact a dependent variable.
Usage
An economist could employ this method to investigate how factors like family income, education level, and local unemployment rates collectively influence consumer spending. For example, a study may reveal that a 1% increase in income correlates with a 0.5% rise in spending.
3. Polynomial Regression
When variables have a non-linear relationship, polynomial regression fits a curved line to the data.
Application
In stock market predictions, where price trends can fluctuate dramatically, polynomial regression might yield better forecasts than linear models. For instance, it could accurately map how stock prices behave differently over several months, thus improving investment strategies.
4. Logistic Regression
Primarily used for binary outcomes, logistic regression can also support trend analysis by predicting the likelihood of a specific event.
Illustration
Imagine a study exploring whether specific weather patterns contribute to crop failures. Logistic regression can predict the chance of failure based on factors like rainfall and temperature, helping farmers make better planting decisions.
The Role of Regression in Financial Forecasting
In the finance sector, regression analysis is essential for data-driven insights that guide investment and risk management.
Forecasting Market Trends
Financial analysts utilize regression techniques to model market trends and facilitate predictions. For instance, by analyzing the historical correlations between interest rates and stock prices, an analyst could predict that a 1% rise in interest rates may lead to a 15% drop in stock prices.
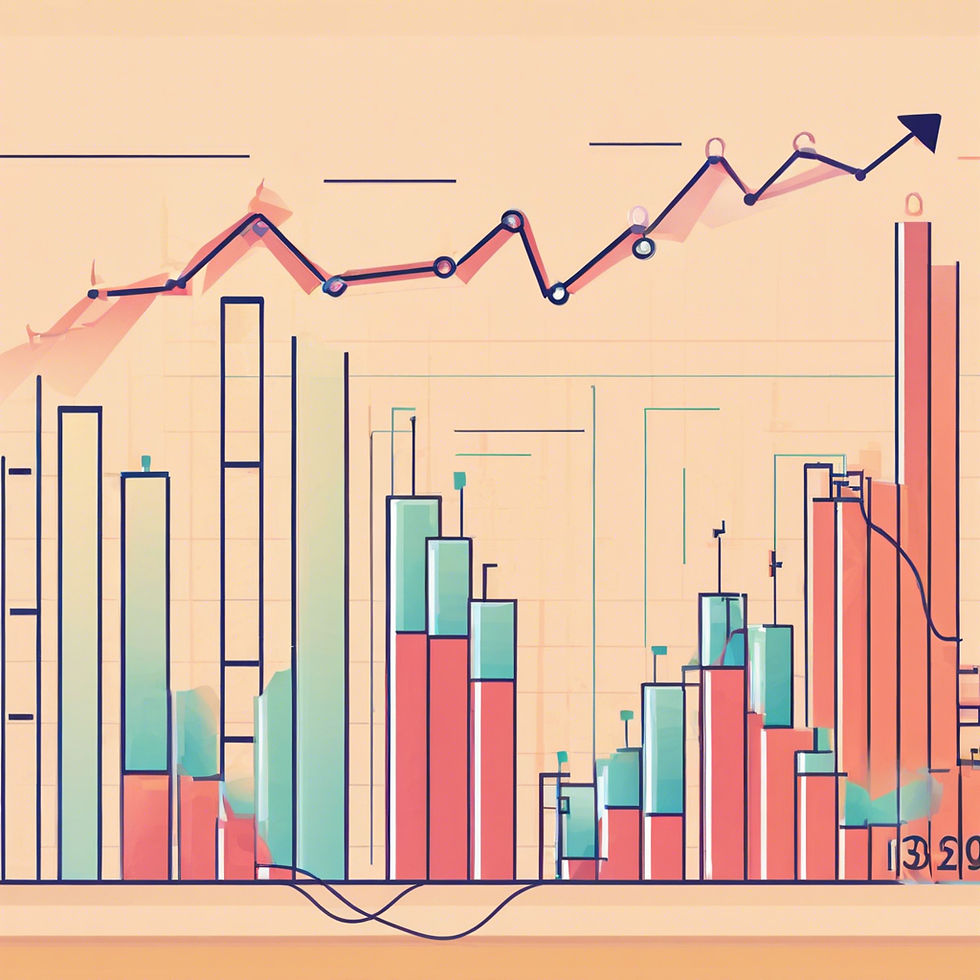
Risk Assessment
Regression analysis also aids in risk assessment. By quantifying how risk factors impact financial outcomes, businesses can identify potential hazards and develop mitigation strategies.
For example, a bank might use multiple regression to evaluate how economic indicators like unemployment rates and inflation influence loan default rates, enabling them to make more informed lending decisions.
Regression in Environmental Studies
Regression analysis is equally vital in environmental studies, providing critical insights into climate change and conservation efforts.
Climate Change Predictions
Researchers frequently employ regression to examine the link between greenhouse gas emissions and global temperatures. By analyzing decades of data, scientists can predict that a 2% increase in carbon emissions could result in up to a 1.5°C rise in global temperatures.
Conservation Efforts
Regression models help identify key factors impacting biodiversity, aiding conservationists in their work. For instance, by analyzing how habitat loss correlates with the decline of specific species, conservationists can prioritize efforts in areas where protection is most needed.
Importance of Data Quality in Regression Analysis
The effectiveness of regression analysis hinges on the quality of the data used. Inaccurate or biased data can lead to misleading results and misinformed strategies.
Data Preparation Steps
To ensure reliability in regression outcomes, careful data preparation is essential. Key steps include:
Data Cleaning: Correcting inaccuracies and removing outliers that may distort the results.
Variable Selection: Identifying relevant variables that accurately represent the studied relationships.
Normality Checks: Ensuring the data meets the required assumptions for robust regression analysis.
By focusing on data quality, organizations can improve the reliability of their trend analyses and subsequent decision-making.
Challenges in Regression Analysis
Despite its advantages, regression analysis comes with challenges analysts must navigate.
Multicollinearity
This occurs when independent variables are highly correlated, which can skew regression results. For example, if both income and education level are included as predictors in a model, it may become difficult to distinguish their individual effects on consumer spending.
Overfitting
Overfitting happens when a model learns the noise in the data rather than the underlying trend. This results in poor performance when applied to new datasets. For instance, a model that fits perfectly to historical sales data may fail to predict future sales accurately.
Assumption Breakdowns
Common assumptions of regression, such as linearity and independence of errors, must hold true for the model to be valid. If these assumptions are violated, the results may be unreliable and necessitate reassessment of the modeling approach.
Awareness of these challenges enables analysts to adopt corrective measures, leading to more robust regression models.
Applications of Regression Analysis in Trend Forecasting
Regression analysis is widely applied across various industries, continually aiding trend forecasting.
Healthcare
In healthcare, regression predicts patient outcomes based on treatments or lifestyle choices. For example, analyzing a dataset on diabetes patients may reveal that adherence to medication regimens increases the chance of better health outcomes by 30%.
Retail
Retail businesses examine sales data against variables like promotions and economic factors. Regression models help them strategize inventory and optimize prices. For instance, a retailer may find that a seasonal sale can boost sales by 40% compared to a normal sales period.
Sports Analytics
Regression techniques are also prevalent in sports analytics to evaluate player performance. For instance, analyzing data on player stats may help teams understand how a player's scoring increases linearly with training hours, aiding contract negotiations and team strategies.
Ethical Considerations in Regression Analysis
With the power of regression analysis comes the responsibility to consider ethical implications.
Data Privacy
Maintaining data privacy and ethical standards in data usage is crucial. Organizations should strive to be transparent about their data collection processes and prioritize user consent to build trust.
Bias in Data
Data used in regression models can carry inherent biases. It's essential to scrutinize data sources and address any biases to ensure fairness, particularly in fields like hiring practices and law enforcement decisions.
The Future of Regression Analysis
As technology evolves, so too does our approach to regression analysis. The integration of artificial intelligence and machine learning can empower regression models to become even more sophisticated. These advancements will offer deeper insights, allowing stakeholders to make more informed and strategic decisions.
In conclusion, regression analysis serves as a cornerstone in trend analysis, offering valuable insights across various fields. By adhering to best practices in data selection and analysis, while remaining vigilant about ethical considerations, we can effectively leverage the potential of regression analysis to navigate the complexities of a data-driven world.
Understanding regression is not just about making predictions; it's about enhancing our understanding of the interconnectedness of various factors, leading to impactful and informed decisions in our daily lives.